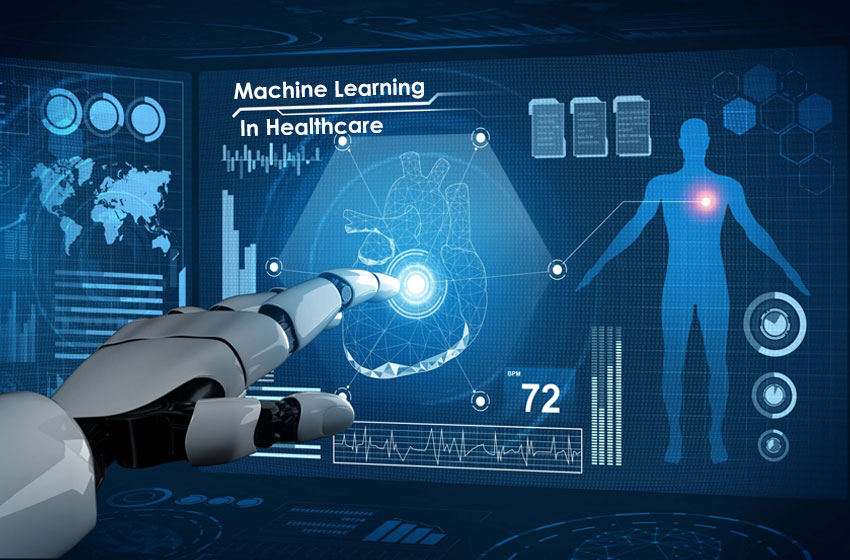
Important Role & Responsibility Of Machine Learning In Healthcare
- New Innovation & Researches Technology
Sophia Silva
- May 4, 2022
- 0
- 9 minutes read
Machine learning is basically a subclass of AI (Artificial Intelligence) Technology, where algorithms process huge sets of data to detect patterns, educate from them, and implement tasks autonomously without being instructed on how to effectively address the issue. In recent years, the broad availability of strong cloud and hardware computing has resulted in a wider adoption of ML. With that respect let us have a look at the role of machine learning in healthcare.
Healthcare is a sector that keeps up with the latest times also. With the portion of data developed for every patient, machine learning algorithms in the healthcare sector have a good amount of potential. So that is no wonder that there are several successful applications of machine learning in the healthcare space presently. Without any further delay, let us learn more about this as there are many machine learning in healthcare companies that are presently working in the company.
Activities That Machine Learning In Healthcare Can Manage
The techniques of machine learning can be applied to solve a wide range of activities. When it is to applications of machine learning in healthcare, these tasks include:
- Classification: machine learning algorithms can support the evaluation and label the form of disease or medical scenario you are dealing with.
- Recommendations: The algorithms of machine learning can provide important medical data without the requirement to actively look for it.
- Clustering: ML and helps to group together the same medical cases to evaluate the patterns and carry forward research in the future.
- Prediction: Utilizing present data and also common trends, ML can make a prediction on how the future scenarios will unfold.
- Anomaly Detection: Employing machine learning and artificial intelligence in healthcare, you can find the elements that stand out from common patterns and evaluate whether they need any actions to be performed
- Automation: Machine learning can manage standard repetitive activities that take too much effort and time from patients and doctors, such as data entry, appointment scheduling, inventory management, and many more.
Opportunities Of Machine Learning In Healthcare
Using machine learning in healthcare for activities that have been stated above can offer a lot of chances for healthcare organizations. First, it enables healthcare professionals to concentrate on patient care rather than spending their time on data search or entry.
The second crucial role of ML in healthcare is the rise of diagnosing accuracy. For instance, machine learning has proven to be 92 percent accurate in anticipating the mortality of COVID-19 patients.
Third, using machine learning in medicine can help to create a more precise treatment plan. A lot of medical scenarios are unique and need a special approach for side effect reduction and effective care. Machine learning algorithms can ease the search for such solutions.
Advantages Of Machine Learning In Healthcare
Using machine learning in healthcare functions can be extremely advantageous to the company. Machine learning was created to deal with large sets of data, and patient files are exactly that, many data points that require thorough organizing and analysis.
Moreover, while a medical professional and a machine learning algorithm will most likely attain the same conclusion depending on the same data set, using ML will get the results much quicker, enabling the treatment to begin earlier.
Another point for using ML techniques in healthcare is discarding human association to some degree, which decreases the chances of human error. This especially worries process automation activities, as tedious routine work is where humans err the most.
Top 5 Examples Of Machine Learning In Healthcare
Now that you have known a lot about machine learning and its implications in healthcare, let us have a look at some of the most effective methods of machine learning in the healthcare sector.
1. Clinical Decision Support Systems
Clinical decision support elements help evaluate large volumes of data to determine a disease, plan on the next treatment stage, evaluate any potential issues, and overall enhance patient care efficiency. CDSS is a powerful element that helps the physician do their job effectively and quickly, and it decreases the chances of getting an incorrect diagnosis or prescribing ineffective treatment.
This utilization of machine learning in medicine has been around in this sector for a while but has become more general in recent years. The reason behind it is the broader acceptance of the EHR system (electronic health record) and the digitalization of several data points, that include medical images.
2. Smart Recordkeeping
Ensuring that all the patient records are updated on a regular basis is challenging, as data entry is an uninteresting task. However, it is also important for effective decision-making and also better patient care.
One of the utilization of machine learning in healthcare is using OCR (optical character recognition) technology on the handwriting of the physicians, making the data entry seamless and fast. This data can then be evaluated by other machine learning elements to enhance decision-making and patient care.
3. Machine Learning in Medical Imaging
For the longest time, medical pictures, such as X-rays, have been analog. This has restricted the use of technology for irregularity identification, overall disease research, and case grouping. Fortunately, the digitalization of the procedure has led to more chances with these forms of data analysis, which include the help of machine learning. And, as per a recent meta-analysis, machine learning algorithms do the responsibility as well as human specialists, with 87.0 percent sensitivity and 92.5 percent specificity for the deep learning algorithms and also 86.4 percent sensitivity and 90.5 percent specificity in the case of human physicians.
4. Personalized Medicine
What makes healthcare such a complicated and resource-heavy field is that every scenario has its specifics. People often carry a sleuth of conditions that need simultaneous treatment. So, complicated decisions must be made to build an effective treatment plan that accounts for drug interactions and decreases potential side effects.
5. Data Collection
One of the most crucial responsibilities of a physician is to assemble the history of a patient properly. This can at times be challenging, as the patient is not a specialist and does not know which data is appropriate to disclose.
Using machine learning in healthcare sector, healthcare professionals can evaluate the most appropriate questions they should ask a patient depending on several indicators. This will help gather relevant data and, at the same time, receive a prediction of the most likely scenario.
Final Thoughts
Machine learning already has many effective use cases in the healthcare sector, but it also has the ability to do a lot more. Along with patient diagnostics and also treatment development, it can be used to enhance medical care, anticipate outcomes, and even help with surgeries.
While machine learning in healthcare has a lot of potentials, it also arrives with several challenges, such as building physician-friendly products, healthcare data quality, and gathering a big team of data experts. There also are several ethical issues that include accountability and patient safety. Despite a few challenges, the advantages of ML and AI in healthcare outweigh them prominently. If you plan to harness ML for your healthcare institution, you should proceed without thinking further. This article will help you as a guide on the introduction to machine learning in healthcare.